Resources
That's Fresh! Newsletter
Read a selection of our past issues.
- Clearbox AI Goes Open Source – Meet Synthetic Kit! 🚀Our Open Source journey has begun!January 27, 2025
- Unwrap Our Latest News Before Christmas 🎁Catch our latest AI series and learn about groundbreaking tech developmentsDecember 18, 2024
- SURE, an open-source library for synthetic data evaluation🌎 Discover our open-source project!November 27, 2024
- 🙌 NumPy 2.0 is almost out!And: Our new data preprocessor with Polars | Interview with S2E at Italy Insurance ForumJune 5, 2024
- 😮 What a month for new LLMs!And: Datacamp webinar with ShaliniMay 22, 2024
- ✨ GenAI true value lies beyond operational enhancementsAnd: The Future of Data Protection | New updates about AI ActApril 24, 2024
- 👁 What are 1-bit Large Language Models?And: Linkedin Live about AI Act | Mastercard's Country Manager interviewed our CEOMarch 6, 2024
- LLaMAntino - Effective Text Generation in ItalianAnd: Creating train and test datasets | Use case: Detecting money muling with the help of synthetic dataFebruary 21, 2024
- 🗞️ The NY Times sues OpenAI and MicrosoftAnd: Can AI work with little data? | La Stampa: AI means developmentJanuary 10, 2024
- Synthetic Data 101 🚨And: Why synthetic data? | New project with Poste ItalianeNovember 8, 2023
- How easy is it for LLM to infer sensitive information?And: Why is data sharing important? | Our new partnership with S2EOctober 25, 2023
- Have you heard of Pythia?And: Data augmentation tutorial | Did you say AI apocalypse?August 30, 2023
- Google's answer to ChatGPTAnd: Generating synthetic data within relational databases. Let's meet at WAICF!February 8, 2023
- Understanding ChatGPT betterAnd: How to deal with imbalanced data. More about our productDecember 14, 2022
- A curated list of failed ML projectsAnd: How to build a data strategy. Clearbox AI and Bearing Point partnership.November 16, 2022
- Our open source library is now on GitHubAnd: Clearbox AI on Cybernews.June 22, 2022
- Discovering DagsterAnd: Quantifying privacy risks. Use case: a synthetic data sandbox to freely share data.June 8, 2022
- Can interaction data be fully anonymized?And: Synthetic Data for privacy preservation: understanding privacy risks. Discover our Enterprise solution.April 6, 2022
- What are GFlow nets?And: Improve models with Synthetic Data. Use case: augment financial time series.March 16, 2022
- The European Commission selected us for Women TechEU pilot project!And: What is Synthetic Data. The new Synthetic Data platform.March 09, 2022
- The EDPS on Synthetic DataAnd: From raw to good quality data. Changelogs: now you can upload unlabeled datasets.February 23, 2022
- 2022 Gartner’s Technology TrendsAnd: How to harness the power of AI in companies. Changelogs: new metrics available for your synthetic dataset.February 09, 2022
FROM THE AI WORLD
I've recently stumbled into this nicely curated repository containing a list of machine learning projects that failed miserably. This list includes different model families and domains and links to relevant news sources. You might have heard about these ML incidents individually while reading the news; however, having a repository collecting and updating them is an excellent idea. I was familiar with the most famous ones, such as the Apple Credit Card or Zillow projects; nevertheless, I was happy to learn about less-known ones.
ML project failures can arise for different reasons, ranging from poor problem definition to problems with the data used to train the model. The data aspect will become more prevalent as large-scale NLP and computer vision models use massive datasets for training. These datasets are becoming increasingly difficult to clean and curate, so it is equally challenging to control what models learn. As they say, garbage in, garbage out!
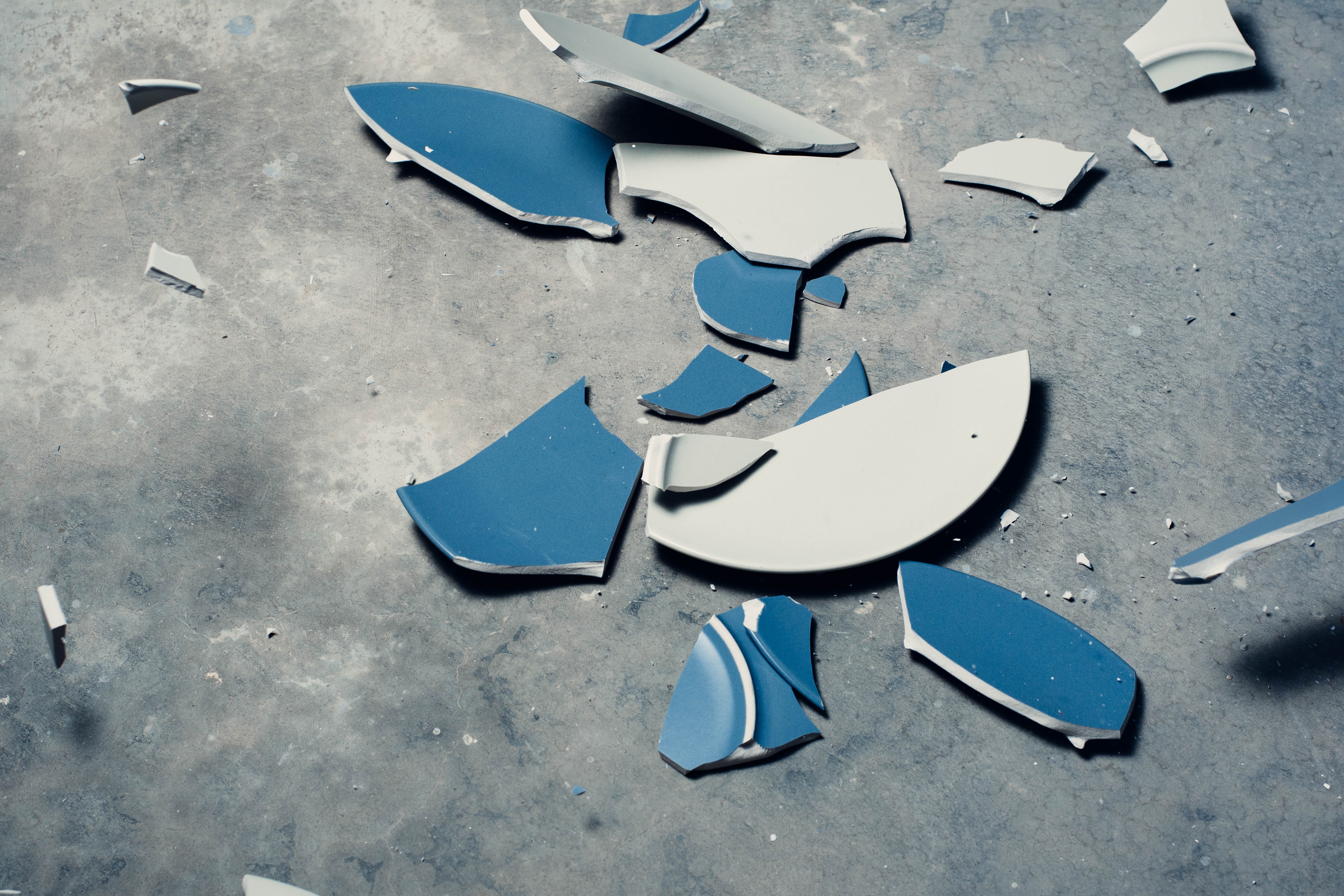
ML projects epic fails
Despite the potential, ML projects may fail. In this Github library curated by Kenneth Leungty you'll find real world examples from which we can learn a lot.
INTERVIEW
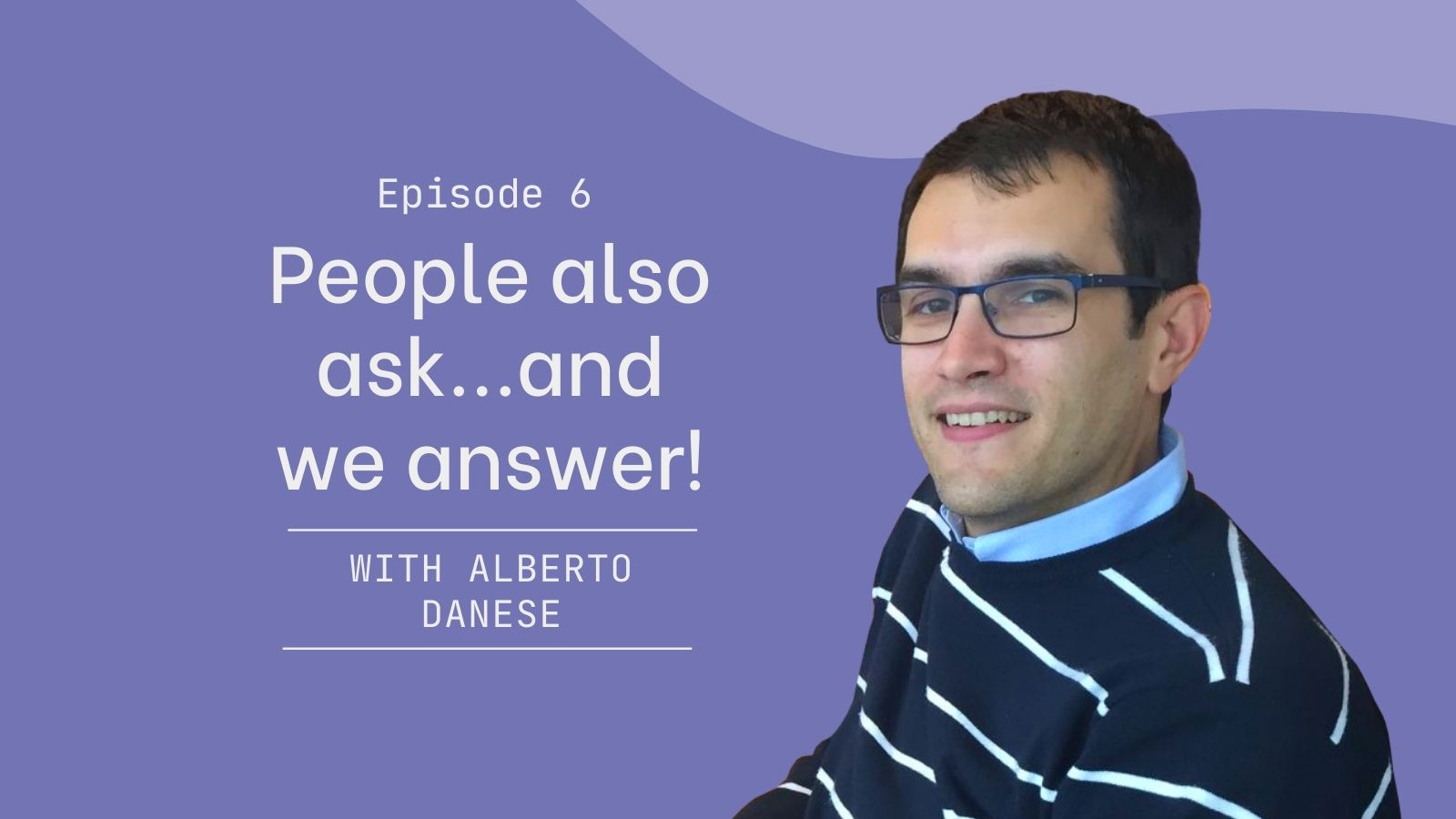
How to build a data strategy
In one of our latest episodes of "People Also Ask...and we answer!" we interviewed Alberto Danese, Head of Data at Nexi, and discussed about data strategies.
HOT FROM THE PRESS
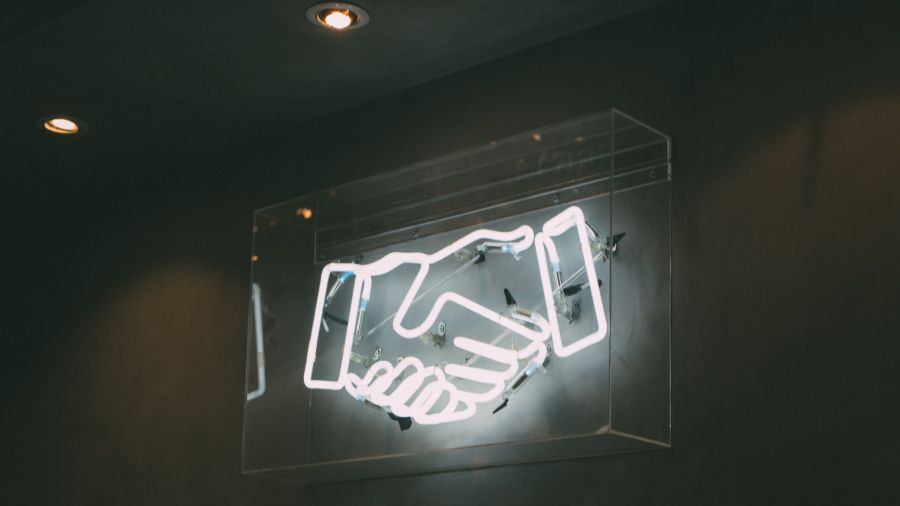
Clearbox AI and BearingPoint
We recently started a partnership with BearingPoint, leader in the technology consulting, for fraud detection and help fintech institutions. (Lang: 🇮🇹)
WEEKLY MEME
Your pals may also like...
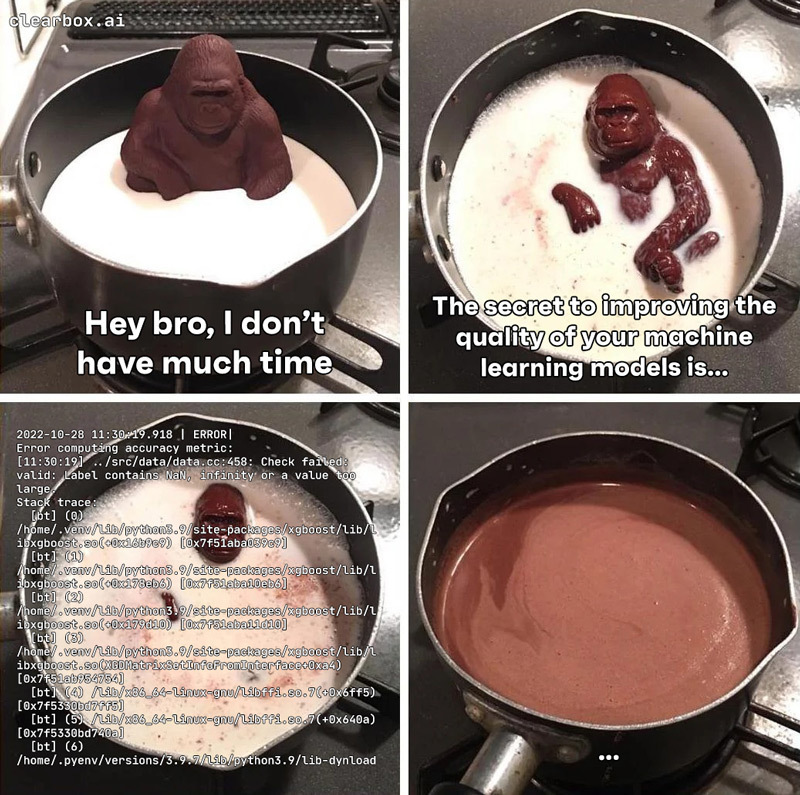