Resources
What is Synthetic Data?
Synthetic Data is obtained by generating artificial data that
incorporates an original dataset's
statistical properties and distributions, thus reflecting
real-world data. This data augmentation technique can be used instead of
or in addition to original data to improve AI and Analytics projects and
to solve different data-related problems.
"By 2024, 60% of the data used for the development of AI and analytics
projects will be synthetically generated."
Gartner
Why Synthetic Data?
As companies start to accelerate their AI adoption within their business
processes, they face escalating challenges as they take stock of the
data required for the AI models.
These issues are often related to data governance aspects like access
and sharing of privacy sensitive data and related data retention
problems or sometimes data quality is not good enough to guarantee a
successful outcome. Compared to other anonymization techniques, or
pseudonymised data, synthetic generation joins utility and privacy
goals. Its risk of reidentification is very low and it reduces AI
projects costs related to data collection and labeling.
Protect data and preserve its privacy
Synthetic generation improves de-identification and creates data sandboxes to share data inside and outside your organisation easily.
Augment data to unlock its most value
Synthetically generated data mitigates data scarcity and improves ML models’ generalisation by solving imbalance problems.
Take a step forward towards AI fairness
Synthetization is helpful to fix possible bias that lies within the data and ensure a more inclusive AI application.
Improve and automate software testing
Synthetic Data lays the foundation for safer and better testing, for example in data migration cases or as test data generation.
Find out more:
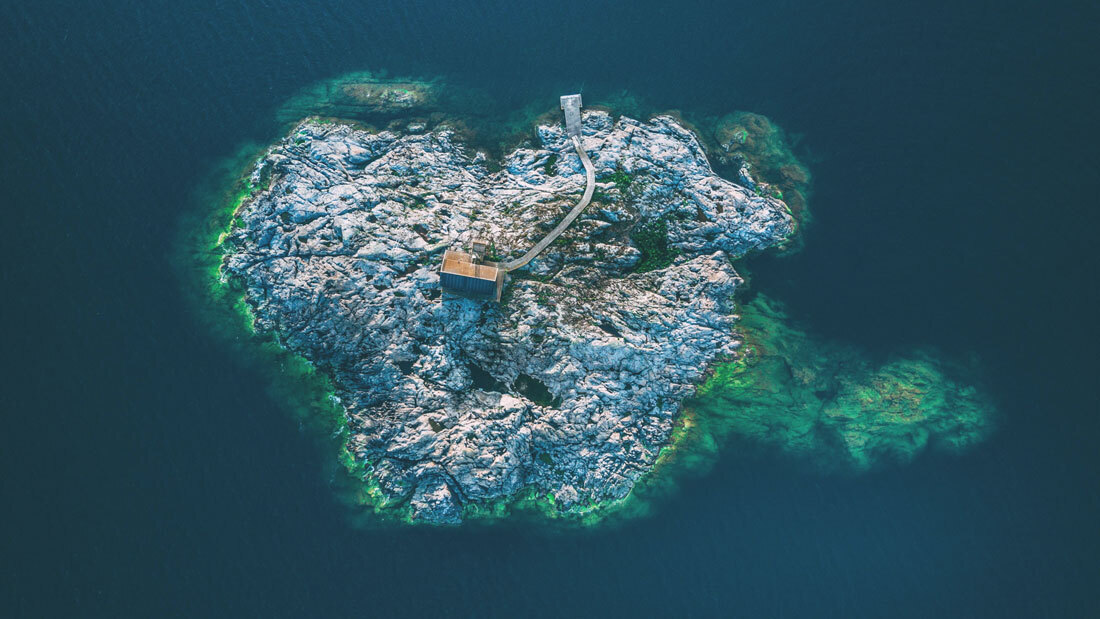
Andrea Minieri 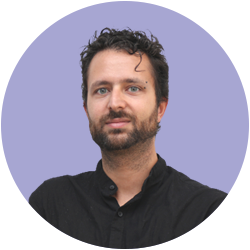
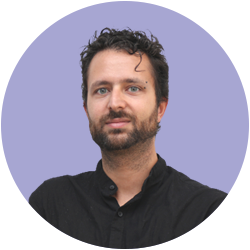
April 5, 2022
Synthetic data for privacy preservation - Part 1
By Andrea Minieri
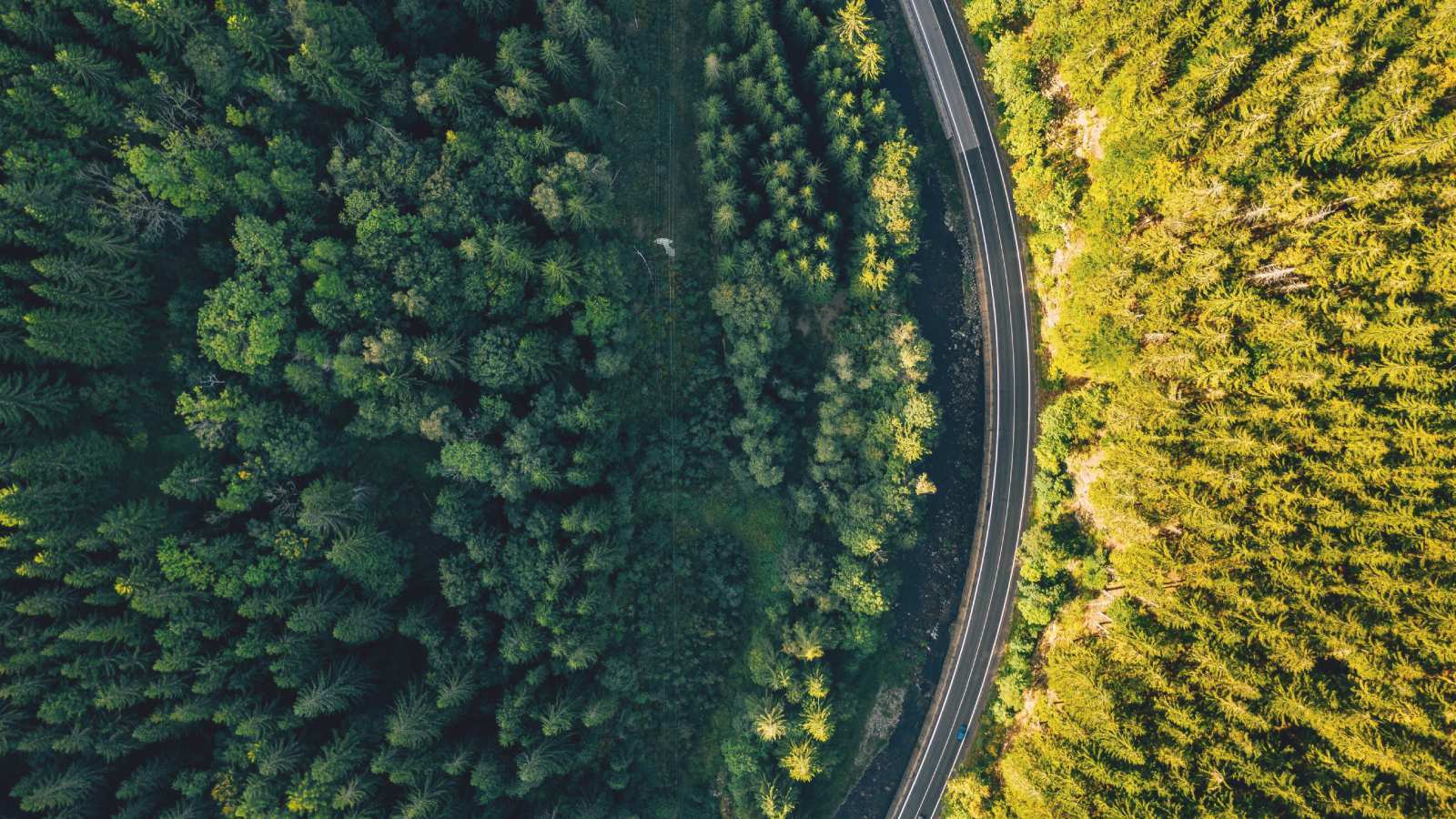
Shalini Kurapati 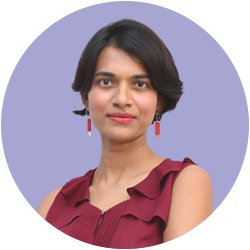
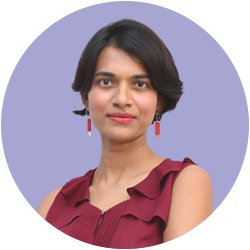
March 8, 2022
What is Synthetic Data?
By Shalini Kurapati
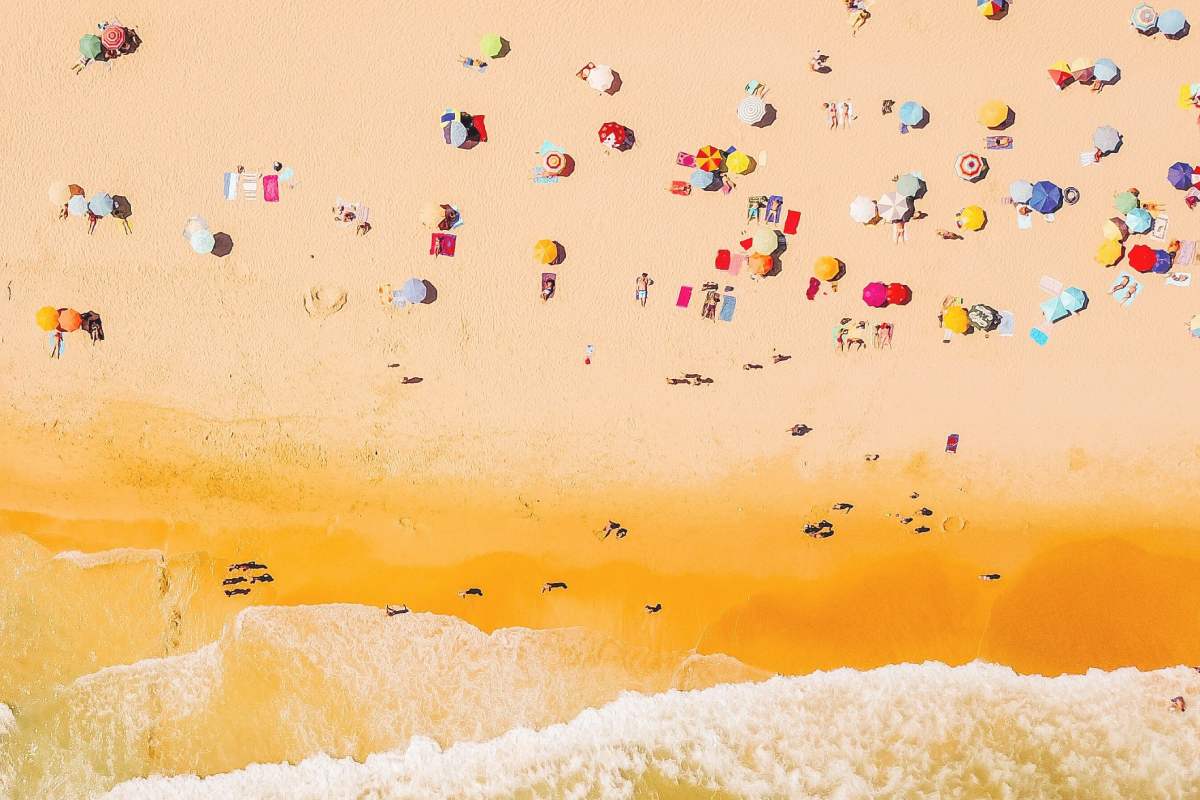
Shalini Kurapati 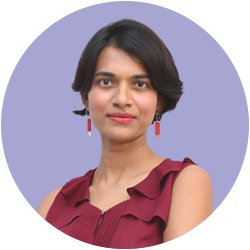
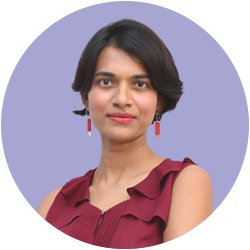
December 9, 2021
You can now evaluate Synthetic data quality on the AI Control Room
By Shalini Kurapati
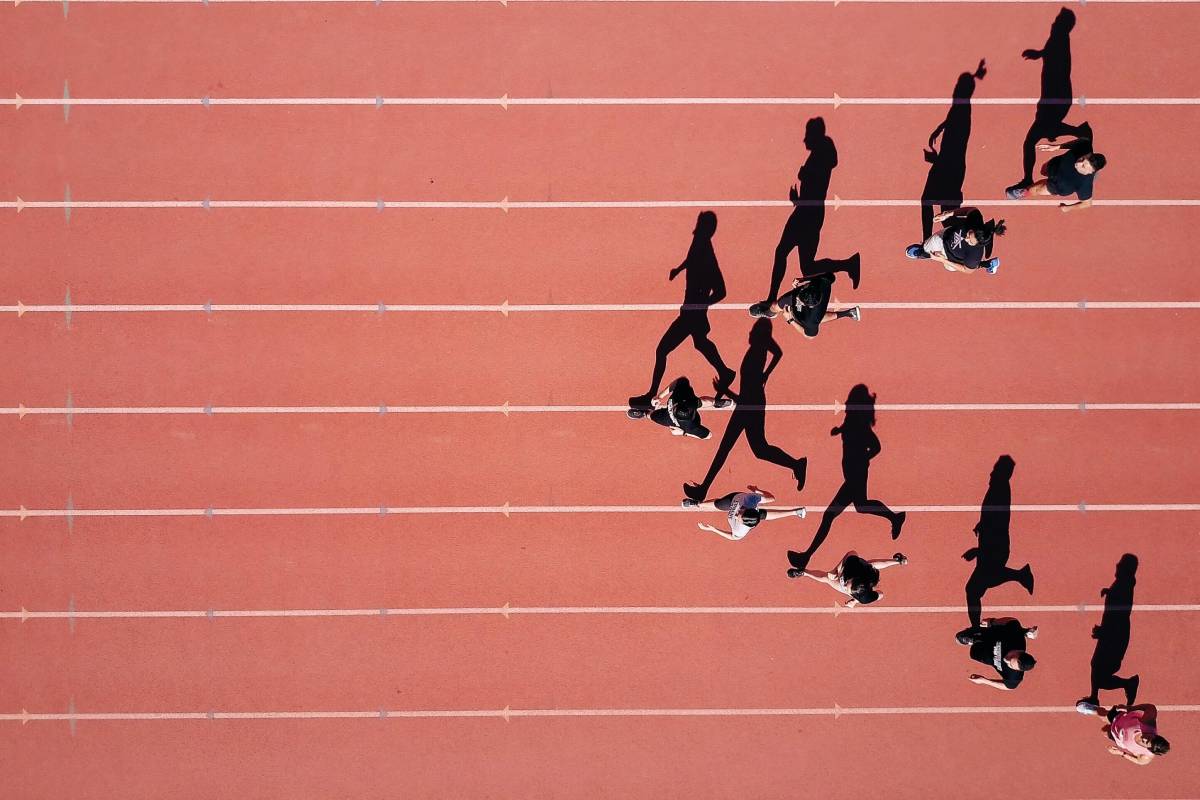
Andrea Minieri 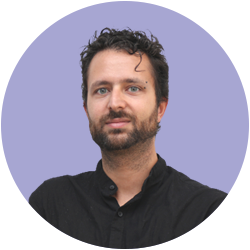
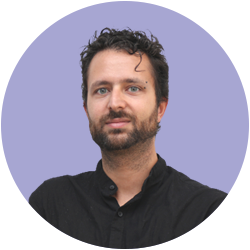
November 30, 2021
How to improve your models with Synthetic Data
By Andrea Minieri
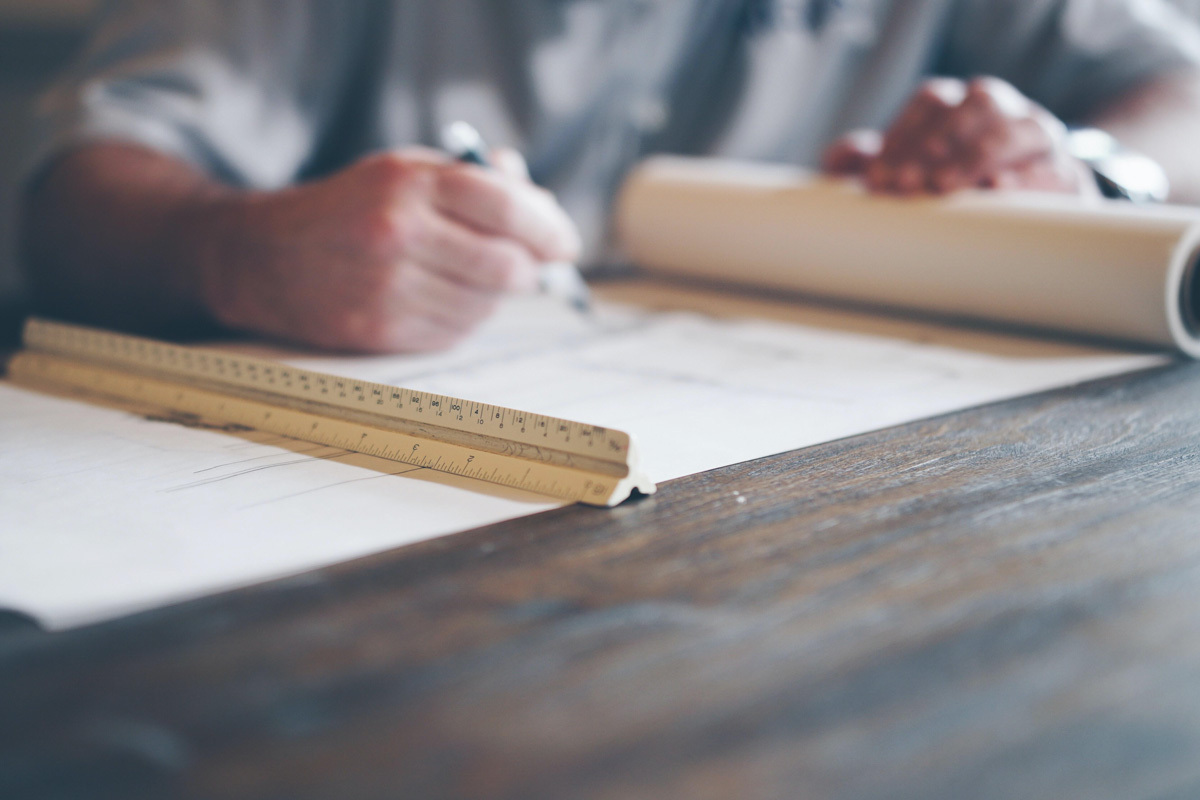
Rabindra Khadka 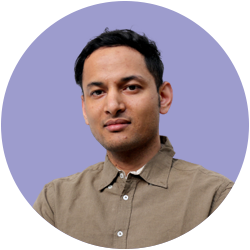
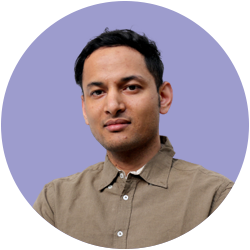
June 9, 2021
How to evaluate the robustness of synthetic data
By Rabindra Khadka
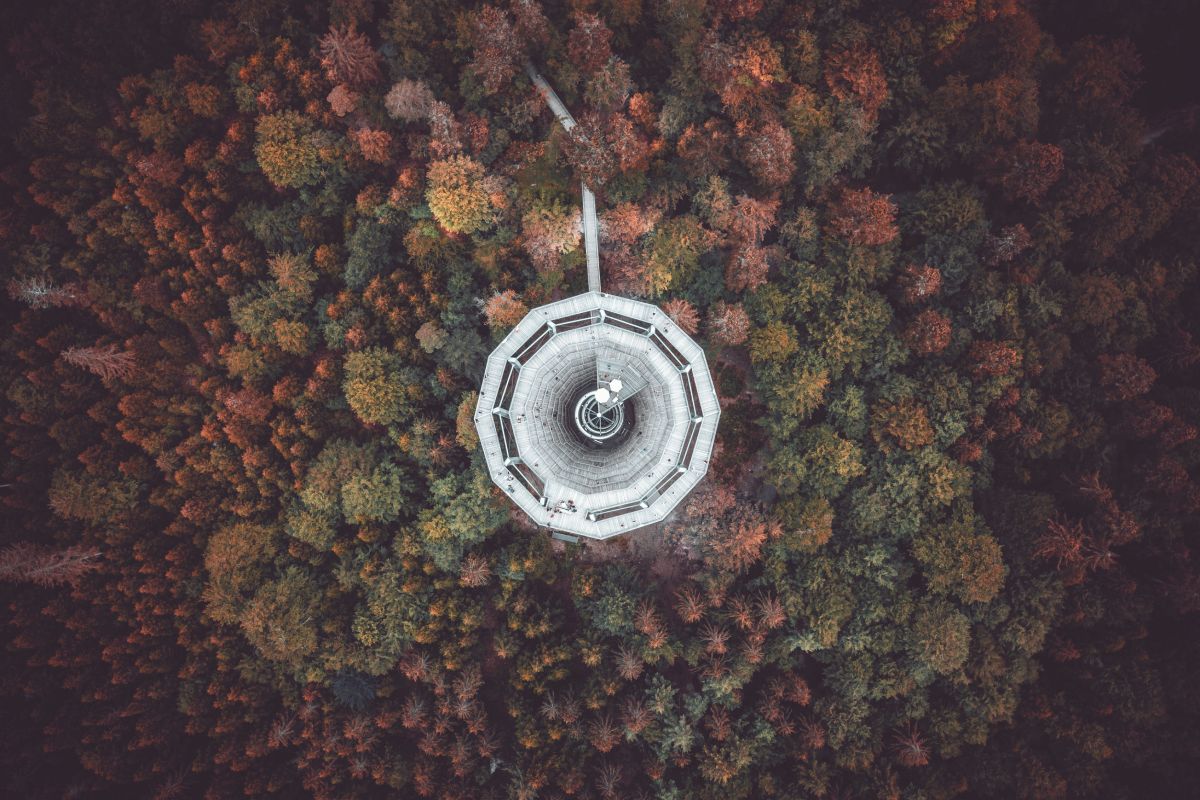
Luca Gilli 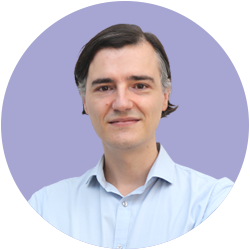
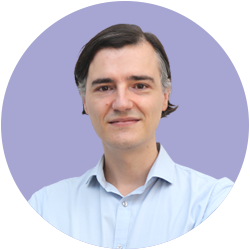
January 13, 2021
Privacy preserving data cloning using generative models
By Luca Gilli