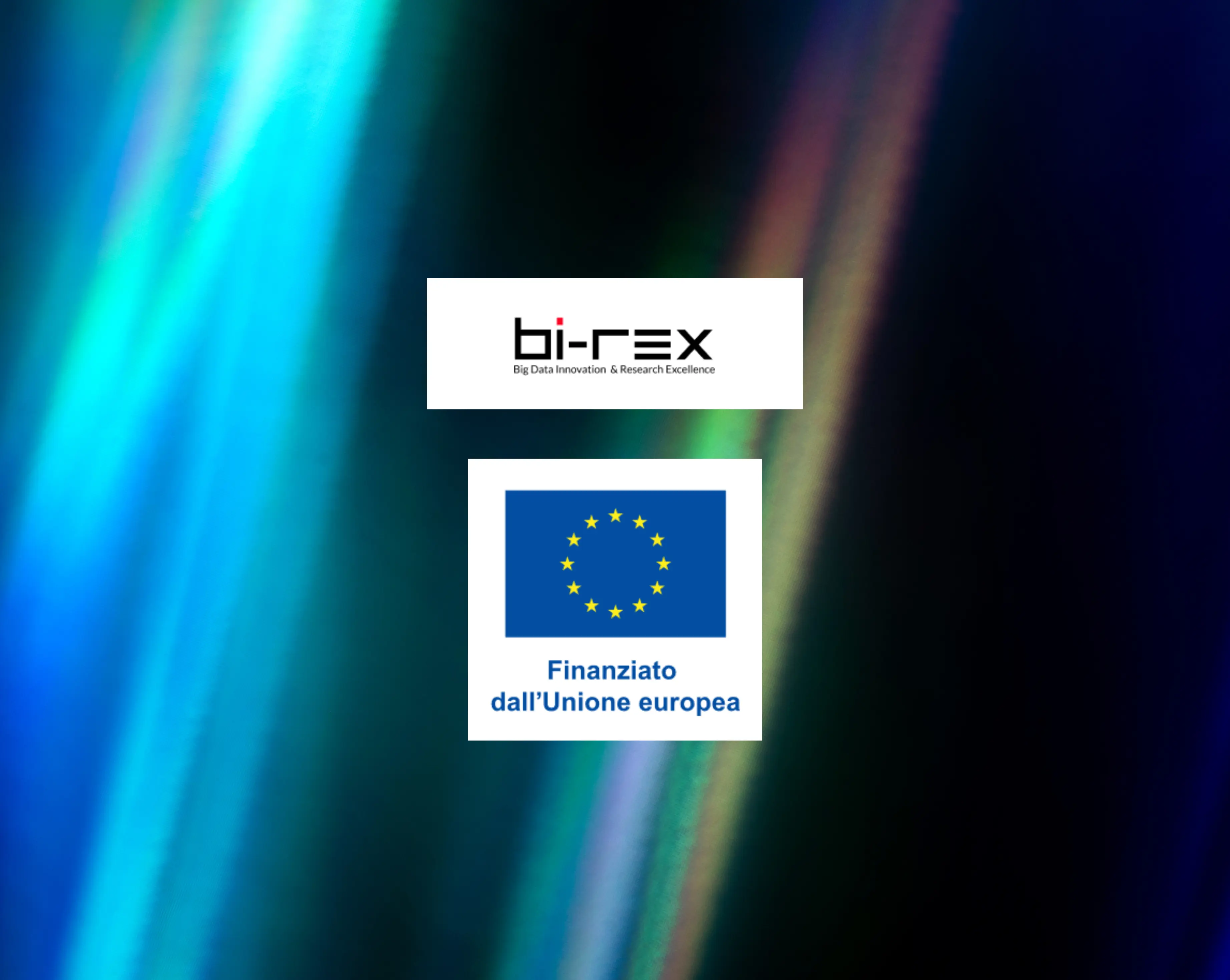
Synthetic data offers numerous advantages to the manufacturing industry, including enhanced machine learning model training and reduced dependency on real-world data collection, which can be both costly and time-consuming. By leveraging synthetic data, we can simulate a wide range of scenarios, allowing for more robust testing and validation of manufacturing processes. This approach leads to greater innovation and efficiency, ultimately driving the industry forward.
Harnessing the power of synthetic data in the manufacturing sector
We are excited to announce our participation as synthetic data providers in the new Next Generation Analytics for Manufacturing (NGA4M) project. This initiative, co-financed by the BI-REX Competence Center (one of the eight national Competence Centers established by the Ministry of Economic Development under the government's Industry 4.0 plan) and the Ministry of Enterprises and Made in Italy, will see Clearbox AI partnering with Bonfiglioli, EMAG SU, and MEP—three leaders in the metal-mechanical manufacturing sector—to unlock the potential of their data using synthetic data.
During the exploration phase, we will analyze data from our partners and deliver custom solutions tailored to their specific needs. Our goal is to help these companies harness the power of synthetic data to improve their operational efficiency, optimize production processes, and gain deeper insights from their data. This collaboration represents a significant step towards a more data-driven future for the manufacturing industry.
Practical applications of synthetic data in the manufacturing industry
Reducing data collection time
In production lines with programmable machines, software updates approximately occur once a year. Each time the program is changed, previously collected data becomes obsolete. Consequently, data scientists have a limited six-month window to gather sufficient data and another six months to analyze it before the next update.
Synthetic data can significantly streamline this process.
Instead of waiting six months to gather enough data, it can collect data for just one month and then generate additional synthetic data based on that initial dataset. This approach allows them to amass a large volume of data in a much shorter time frame, providing more time to analyze the data and derive valuable insights before the next program update.
Breakage prediction
In metal-mechanical production lines, maintaining high-quality control standards is crucial. A breakage rate of one piece per 10,000 (0.01%) is considered average. In well-controlled manufacturing environments, the goal is to achieve a breakage rate of one piece per 100,000 (0.001%). In highly optimized sectors such as aerospace, medical device manufacturing, or high-precision engineering, defect rates can be even lower, approaching near-zero levels.
Improving the breakage rate involves conducting comprehensive reliability studies and implementing Statistical Process Control (SPC). SPC methodology uses statistical tools to monitor and control a process, ensuring it operates at its fullest potential. By analyzing process data, SPC provides accurate insights into issues and identifies areas for improvement, ultimately enhancing product quality and reducing defects.
However, studying breakage phenomena in highly efficient manufacturing environments presents significant challenges. When defect rates are as low as 0.01% or even 0.001%, the data available for analysis is extremely limited. This scarcity of breakage data makes it difficult to identify patterns and root causes, hindering efforts to further reduce defect rates.
Synthetic data can address this challenge.
By simulating additional breakage records, synthetic data effectively balances the dataset. This augmentation allows for more robust analysis and model training. When breakage records are sparse, machine learning models struggle to learn effectively. By generating synthetic breakage data, we can provide the models with enough examples to improve their accuracy and reliability. This, in turn, enables more precise identification of breakage patterns and potential causes, facilitating proactive measures to prevent defects.
Conclusion
In summary, synthetic data offers significant benefits to the manufacturing industry:
- Instead of waiting months to collect sufficient real-world data, manufacturers can quickly generate synthetic datasets, accelerating the analysis and implementation of improvements. This approach not only saves time but also allows for continuous optimization of the production process.
- While achieving low defect rates in metal-mechanical production lines is a sign of efficiency and quality, it poses challenges for data analysis and reliability studies. Synthetic data provides a powerful solution by augmenting scarce breakage records, enabling more effective use of machine learning models and statistical analysis. This enhances the ability to identify and address defects, leading to improved product quality and more efficient manufacturing processes.
This collaborative journey in the manufacturing industry is an adventure we are thrilled to begin. Here, the innovative application of synthetic data promises to revolutionize processes and drive efficiency.
We look forward to leveraging the power of synthetic data to unlock new opportunities, enhance productivity, and propel the manufacturing industry into a future of unparalleled innovation and success.
Stay tuned for finding out the results of this project!
Tags:
news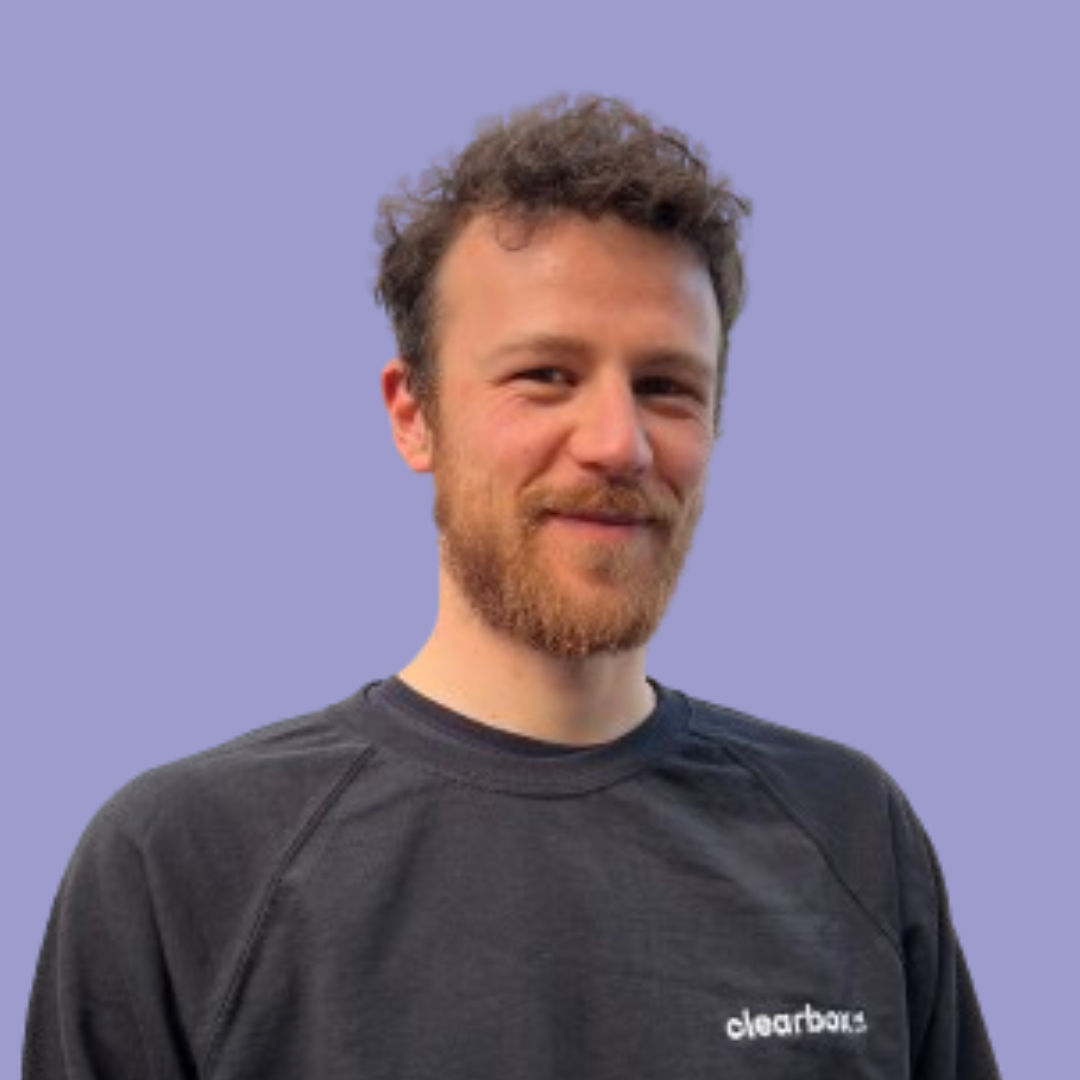