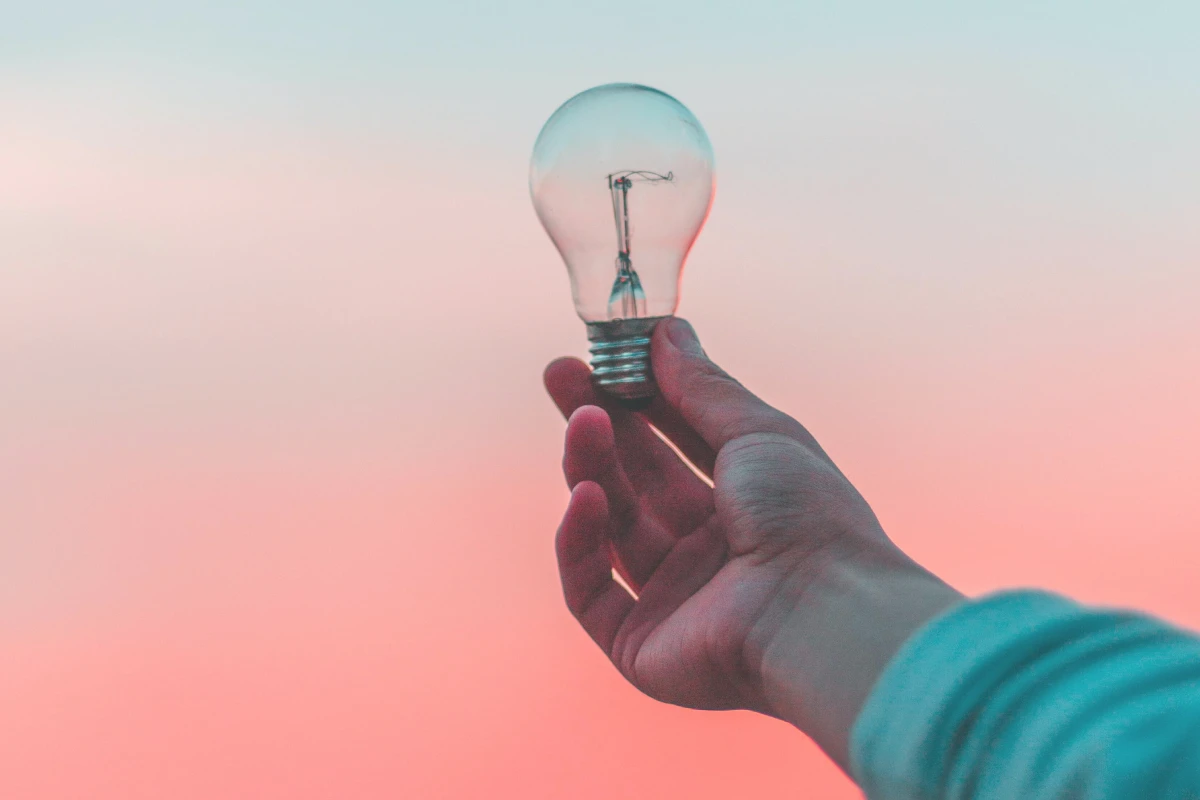
A few weeks ago, I was pleased to be featured as a speaker in the Data Phoenix webinar titled 'The promising role of synthetic data to enable responsible innovation'. This interview is part of 'The A-Z of data' charity AI webinar series, which supports the Ukrainian cause through donations.
Me and Dmitry Spodarets, the founder of Data Phoenix, started the discussion from the basics. What synthetic data is, what its applications are and why it is such a hype nowadays. You can find all about it in one of our previous blog posts. The main focus of the webinar revolved around the concept of responsible innovation related to synthetic data.
Synthetic data for responsible innovation
Oftentimes, people think about synthetic data only as a privacy preserving technology. If we look at 2022 Gartner’s Hype Cycle for Artificial Intelligence, synthetic data takes a pole position in the curve of expectations. It’s at the intersection of Data-Centric AI and Human-Centric AI because of its potential to enhance data quality towards responsible, ethical and secure innovation.
The data side of AI algorithmic discrimination
One of the interesting aspects of generative AI is the lesser-known side of synthetic data, which is that of bias mitigation. You might ask yourself if synthetic data propagates prejudice when it originates from a biased dataset. That’s a fair concern. But there are ways to turn the coin and be aware of this aspect, understand its properties, detect imbalances and mitigate possible bias using synthetic data.
We are all too familiar with articles about biased AI algorithms, including incidents from some of the largest companies in the world. One example is Amazon’s recruiting tool which showed bias against women even when CVs were anonymized. The algorithm understood the gender of the applicants based on their hobbies or the school they went to, and women were discriminated against in the screening process. That is partly because, historically, men belonged more in the workforce than women. That’s a good example of historical bias in datasets.
However, bias in data is not just about gender. It could be related to aspects like ethnicity, and background because datasets often do not sufficiently represent these groups and nuances related to intersectionality.
These biases don't just appear in the algorithms. On the contrary, they come from us, for starters the data we collect, annotate and feed them.
For example, according to image 1, you can see that the symptoms of a heart attack seem to be very different between men and women. This is because many medical studies are historically based on men rather than women, so most of the data that has been used for diagnosing or predicting diseases was focused just on a part of the population.
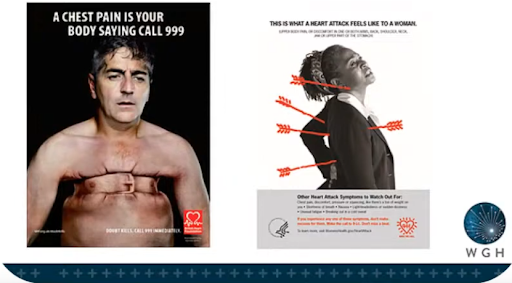
Of course, this is just a dramatic example to show the critical impact that biased algorithms can have even on life and death situations.
When we think of a machine learning pipeline process, bias can originate from the dataset collection phase, its development and labeling. Then, the data feeds into the model and the outputs will also be influenced by the way we build the models, for example in terms of inclusion and explainability. The level of bias we should expect from a model depends on its application as well. For instance, if we use it for the production lines of a factory, bias is not necessarily a big issue. However, when the models are used to make decisions that impact people's lives, the hazards of bias are serious (see image 2).
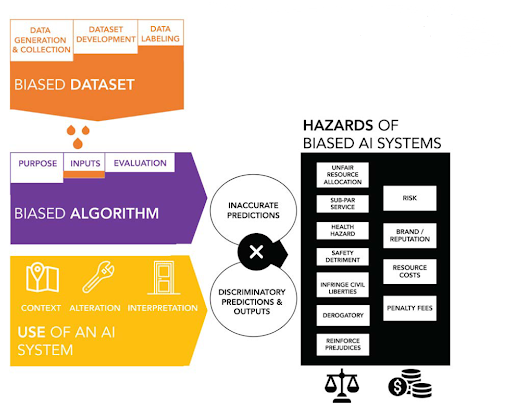
We can broadly classify bias into three categories:
- Systemic bias: generated by historical, societal or institutional data;
- Human bias: individual discriminations based on behaviors, human reporting, rankings, user interactions, or group prejudices;
- Statistical/computational bias: originated from the use and interpretation of ML models, evaluation and more.
Today we will talk about the last one, which may affect data directly. However, we should not forget that statistical and computation biases are just the tip of the iceberg because they come from a much deeper system of societal and human biases rooted in years of history.
Bias mitigation and AI fairness with synthetic data
Now, let's get down to business. How can we measure the fairness level of data in practice? You might even question if the two words fairness and metrics can be used in the same phrase at all. A perfect oxymoron don’t you think? Nevertheless, fairness metrics can be useful as a starting framework to detect and mitigate bias. First of all, I recommend the ‘Fairness and Machine Learning - Limitations and opportunities’ book by Solon Barocas, Moritz Hardt and Arvind Narayanan. It's a free book that allows you to better understand the notions to evaluate bias in your data.
Two metrics that I want to mention when talking about measuring fairness are:
- Equalised odds: are the predictions of my model independent from sensitive features? Are they equal across different groups?
- Equal opportunity: do the conditional expectations remain the same across groups?
For example, let’s take a dataset. We can work on toy datasets you can easily find on Kaggle, like Adult Census Income (image 3).
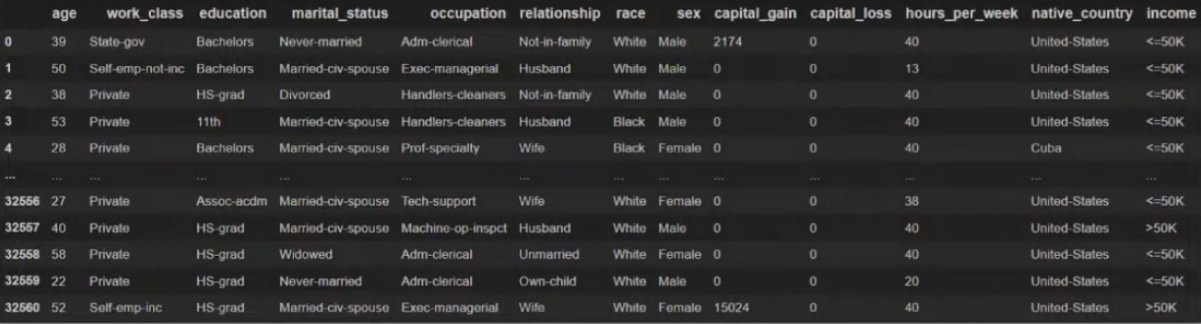
In this case, the model has to predict the income of a certain individual. Once we distinguish the data slices that are useful for us and make the predictions, we can see that the TPR (True Positive Rate) of the different clusters is much higher for men, and after the age of 65 it becomes 0 for females (image 4).
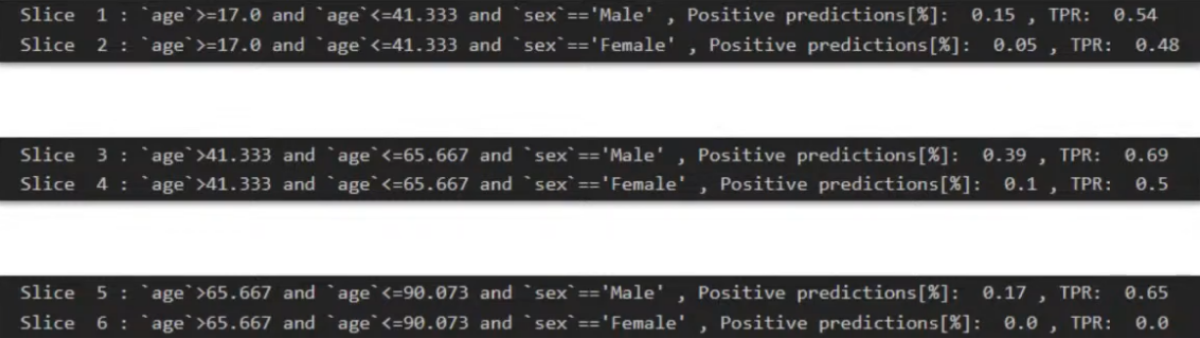
In this example, we can improve fairness metrics by creating synthetic data points to populate specific data slices with positive examples. We can augment the dataset with synthetic data for high-earning women within the 42-90 age range. In the following table, you can see the changes in the metrics once we improved the original information with the newly generated data.
Slice # | Original dataset | Augmented dataset | ||
---|---|---|---|---|
% Positive | TPR | % Positive | TPR | |
Male 17-41 | 15 | 0.54 | 12 | 0.49 |
Female 17-41 | 5 | 0.48 | 5 | 0.4 |
Male 41-65 | 39 | 0.69 | 40 | 0.7 |
Female 41-65 | 10 | 0.5 | 20 | 0.73 |
Male >65 | 17 | 0.65 | 18 | 0.62 |
Female >65 | 0 | 0 | 5 | 0.2 |
In table 2 you can see the results we achieved in terms of model performance.
Original dataset | Augmented dataset | |||||
---|---|---|---|---|---|---|
precision | recall | f1-score | precision | recall | f1-score | |
False | 0.88 | 0.96 | 0.92 | 0.88 | 0.95 | 0.92 |
True | 0.83 | 0.60 | 0.70 | 0.81 | 0.60 | 0.69 |
Weigh. avg | 0.87 | 0.87 | 0.86 | 0.86 | 0.87 | 0.86 |
However, we must underline that this is not always the case. Depending on the synthetic data points you integrate in the original dataset, you will receive different results in terms of fairness or model performance, and this is where the tension might come. Which one of the two do we want to favor? Do we want to have a fairer dataset or do we want to improve the f1 score? The answer is in identifying the trade-off between fairness and hold.out metrics based on the needs of the specific situation.
Conclusions
Fairness can't be fully objective. As William Bruce Cameron says, ‘Not everything that counts can be counted, and not everything that can be counted counts’. This sentence is perfectly suitable for the AI fairness discussion. When you start thinking about implementing fairness metrics in your data pipeline and machine learning processes, you should be aware of a few points:
- What are your/your company’s goals on fairness?
- Consider the involvement of multiple stakeholders and experts to set a framework for unfair biases
- Focus on data quality, because it’s not only important for fairness, but also for the general performance of the model
- Keep testing: try to check with different slices and ways of augmentation
One final suggestion is that you need to remember that there are no perfect ways. Fairness and ethics are by nature not deterministic. Since it’s a complex topic, we shouldn’t be searching for simple answers.
Tags:
interview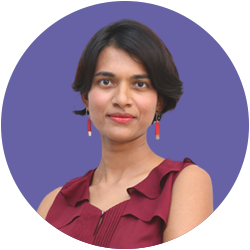