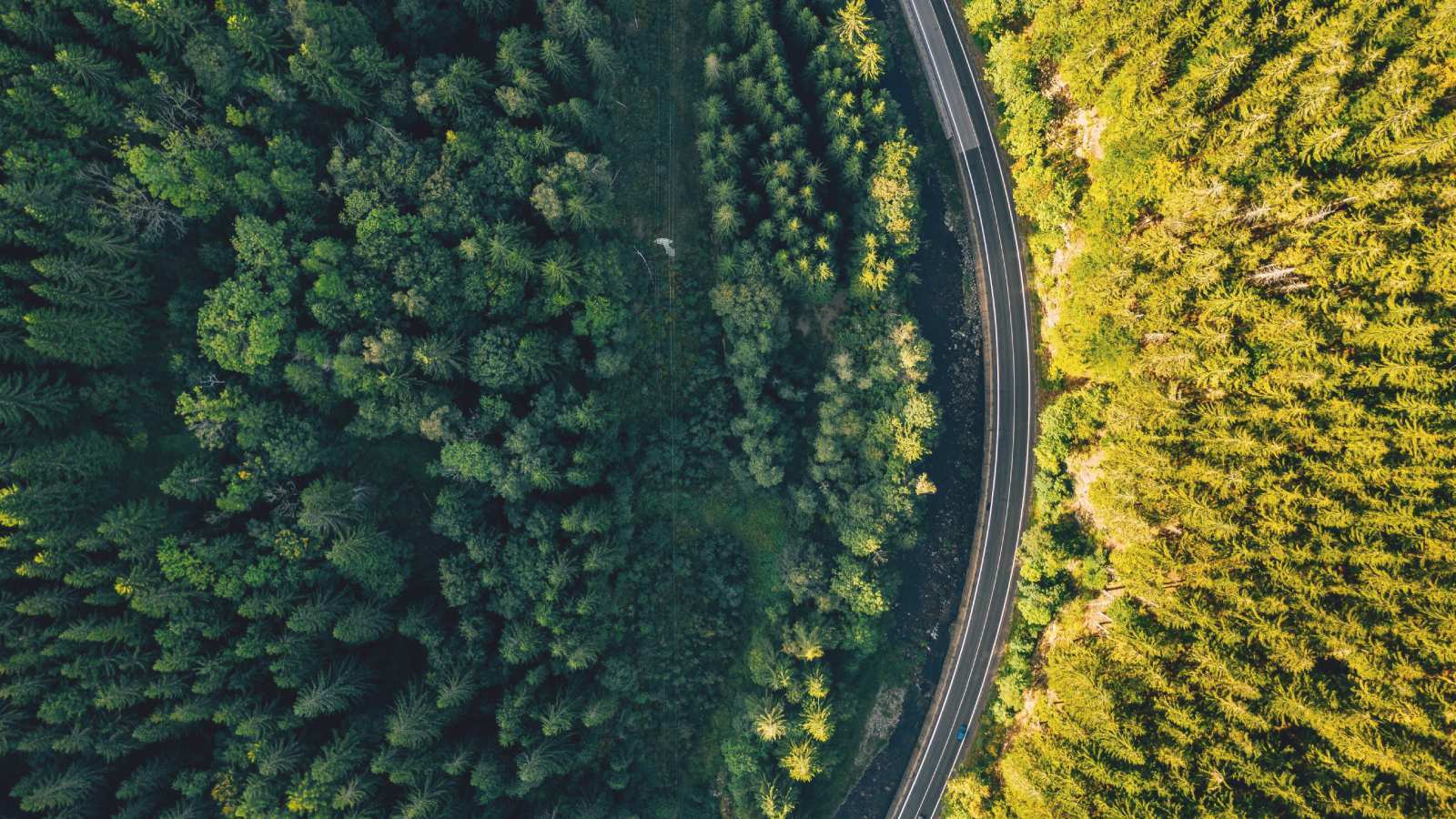
Be it the Gartner hype cycle, the MIT tech review’s breakthrough technology trends, or the EDPS’s opinion piece, the AI sphere is buzzing with talk about the major role of Synthetic Data in solving data scarcity and other AI risks while building AI models.
But what exactly is Synthetic Data?
Synthetic Data is artificially generated, often using AI algorithms upon real-world ‘seed’ data. It has the same statistical properties and predictive power of the real data on which it was generated. It can be a safe proxy for real data since it contains no real personal information for several AI and analytics use cases such as data science/AI projects, test automation and most importantly, privacy preservation.
Synthetic Data in Action
Synthetic Data is becoming increasingly mainstream. Gartner predicts that 60% of AI models will use Synthetic Data in some form or another by 2025. Its recent market trends report also reiterated the importance of generative AI that fuels data generation in its recent hype cycle.
While there are many advantages of Synthetic Data that are driving this trend, not to mention data augmentation, cost-effective and safe data procurement, the main focus these days seems to be on its role in privacy preservation and enhancement. There have already been a few impactful examples of Synthetic Data use cases for privacy preservation. The US Census Bureau employs it in their public datasets and online tools to ‘balance the competing requirements of releasing statistics and protecting privacy’. The NHS in the UK is also experimenting with sharing cancer data for research purposes. Recently, even the office of the European Data Protection Supervisor weighed in on the topic of Synthetic Data.
Data Generation is enticingly complex
So given this widespread interest, application areas and benefits of Synthetic Data, why isn’t everyone generating and using it? For starters, generating Synthetic data is a complex process that requires specialised and advanced AI knowledge, skills tools, and frameworks. Another critical challenge is identifying or sometimes designing sophisticated metrics to evaluate the Synthetic Data based on performance metrics, requirements, and privacy constraints. At Clearbox AI we give utmost importance to Synthetic Data quality and here’s an overview of our evaluation metrics used by our data generation solution.
Another note of caution sounded by organisations using Synthetic Data is related to re-identification of personal data, anonymisation and other unforeseen risks. Here it’s important to highlight how such challenges can be mitigated using a risk quantification approach.
How do we mitigate AI risks with Synthetic Data?
Quantifying the risks can help the users of Synthetic Data make smart choices while dealing with the utility vs privacy conundrum. One such approach is differential privacy. According to NIST: “A differentially private synthetic dataset looks like the original dataset - it has the same schema and attempts to maintain properties of the original dataset (e.g., correlations between attributes) - but it provides a provable privacy guarantee for individuals in the original dataset.”
Another key topic when we talk of risks is that of bias and fairness. Here, Synthetic Data can be a double-edged sword, since there is a chance to replicate or, worse, reinforce bias in the real-world dataset. On the other hand, Synthetic Data can also be a powerful mitigation mechanism to reduce or remove bias. We are currently working on a bias and fairness module in our Synthetic Data generator, and we’ll share more on that in the near future.
Since Synthetic Data will continue to play a significant role in AI and analytics, we think that robust evaluation metrics to ensure data quality and a risk quantification approach to privacy will help companies capitalise on good quality data generation to accelerate responsible innovation.
Stay tuned to find out about privacy preservation metrics for Synthetic Data, which we will share in the coming blog post. In the meantime, take a look at our interview for Cybernews, where we dived into the topic of synthetic data for privacy preservation!
Tags:
blogpost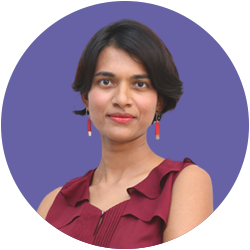