Have you ever run into Google’s ‘People also ask’ section and found the questions you were about to ask? Periodically, we are going to answer the most researched questions about AI and Machine Learning with our guests on Clearbox AI’s new interview series ‘People also Ask...and we answer’. Enjoy!
Artificial Intelligence is constantly changing our lives and improving them. For instance, we can see its power applied in industries every day. Here, AI and machine learning can help to improve processes and business outcomes. To do so, companies need to feed the engine of AI models with their fuel: data. On the one hand, AI models cannot be accurate without using good quality real-world data; on the other hand, this comes with privacy risks. So, how can we prevent and handle privacy risks in AI?
Introducing our guest
Marlon Domingus is the DPO (Data Protection Officer) for the Erasmus University Rotterdam, Netherlands. He specialises in GDPR and speaks about privacy governance, data ethics, privacy by design, privacy engineering, risk management, and privacy audits. He has a background in philosophy. He sees himself as a philosopher in the world of privacy from a European perspective, meaning the relations between the rule of law, human rights and democracy.
What are the privacy issues related to AI?
Marlon: Privacy is a blessing, and a fundamental right. The GDPR protects these rights. We see in practice that most people find it hard to implement the principles defined in the GDPR; thus, the impression occurs is that privacy is complex, but I would like to oppose that idea. In most cases, people think of privacy as data protection, as security stuff, but for privacy, you have to do other things, and have different logic. Now, this is a very interesting question, of course, and because sometimes in AI systems we process personal data, privacy is an issue and we have to consider it. However, I would suggest not to tick the box but to really dive into the topic and what precisely the processing nature of the personal data is and then we’ll see it's more complex than what the logic of the GDPR considers. That is why we need to focus on the specifics of AI. I think we've seen good examples of AI for good, where we can see that big data does indeed help us and we can use it to recognise patterns based on questions that we already ask ourselves. So, that is the good side of AI. I'm not a critic of AI, but I also think we could look at AI for the good, the bad and the ugly. So when things go really wrong, what can we learn from this? I wanted to give a huge example from the Netherlands, where 26,000 innocent families were wrongly accused of social benefits fraud, partially due to a discriminatory algorithm used by the Dutch tax authorities. Citizens were forced to pay back money they didn't owe, and many families were driven into financial ruin, some were torn apart. Others were left with lasting mental health issues, and a remarkable number of children, more than a thousand, were taken from their families. I think that if we apply AI as a black box and say: 'okay. the computer says so, or the algorithm says so', the legal person is lost because the algorithm is more important than the citizens' legal rights. The courts relied more on the algorithms than the individuals, and the distinction between fact and fiction was lost because. The reality of the people was conflicted by what the state said. I think in the end, privacy is about trust. Can we trust markets? Can we trust our government? How do they treat our data? And part of this lies in how they build algorithms and use them for decision-making, how it affects us, and how data is used against us. So, there's the good and the bad, and I think those both matter concerning privacy and AI.
How can we protect privacy while building AI projects?
Shalini: Companies don't want to hear all the nuances because it's complicated, it's messy, and you have to have a holistic view of what it means. So, privacy means a number of rights and not just data protection. Data protection is more the one that you can operationalise; it's easier to implement, so what I would like to extend in terms of privacy risks is whatever you can look into the privacy risk. The most important thing is what is the risk of re-identification. I'm going to use many methods and with my anonymisation techniques, am I able to guarantee that this data set is privacy proof? Or is it good enough? Like Marlon was saying, AI is a complex process where you have an entire cycle of data. You start from acquisition, you transform it, and you store it. Then there is something else happening, and then it goes into the model, and then the model keeps improving, so you have an entire cycle. On each side, you need to have mechanisms where you can test these risks or work in an environment where you can make sure that it's safe for the data subjects you're using, and safe in terms of regulation. Safe in terms of even physical safety or even mental safety, for that matter. Of course, this is the general picture of what we need to consider and we specifically at Clearbox AI we do more of a testbed approach, so we say that, if possible, let's not use real data. From my perspective, starting with Synthetic Data, for example, is a great way to remove already a number of risks that will come along and then slowly try to see at what stage you can include real data also based on the GDPR principles, what is the purpose. Are you using it to test and improve your systems or to share the data? That also depends. Another thing is also the proportionality, so how risky is this. So if it's a very high risk, probably you need to undergo a lot more mechanisms, or maybe you need to implement everything in every stage of your process. But if the risk is relatively low, you can also tailor your needs based on the level of risk. For me, I would start already with the data minimization. Try not to use the actual data; if you have the possibility of training an algorithm with synthetic, start with that.
Is Synthetic Data better or more beneficial than other anonymization techniques in any way?
Shalini: I think the main question here is the principle of privacy versus utility balance: how do we have complete data representing the real world and still protect privacy? This is very hard to achieve because, with most anonymisation techniques, you could also create unnecessary or unwanted effects in your AI models if you remove too much information. So, synthetic could be a very strong contender for this to have a balanced effect between privacy and utility. In terms of direct identification, it's very difficult to directly identify using synthetic. There are some other risks like inference that need to be addressed, but for me, the most significant advantage would be having a high utility with low privacy risk.
How does GDPR affect AI?
Marlon: From a legal point of view, this is going to be very interesting. I also have to mention the e-Privacy regulation, which couples with GDPR, and then the DSA and the DMA, which are another couple with a different logic compared to the GDPR, where there's a more basic and more simplistic allocation of responsibilities of the processing of the data. We have these other regulations called the DGA and the DA, the Digital Governance Act and the Digital Act, created to promote the reuse of data, for instance, for AI purposes. As usual, it's going to be challenging to understand the guidance, so the practical implementation aspects of how these legislations work together – which is in specific cases where two legislations conflict with them –, which one has the higher hierarchy, so which is the lex specialis. We need to decide these things. One of the critical points I also hear in the debates about the AI Act and the DSA is that there's a legal framework. Still, the technical implementation is sometimes based on certain assumptions, which mean investments from organizations to be compliant with these regulations. The GDPR was in place to have a more robust digital single market to have a free flow of data between the countries and the organisations within the EU to promote public-private collaborations, and to have innovation and a stronger internal market. What we see in practice, though, is that people do the checkbox approach, and I think we can expect the same with the AI Act, which is also risk-based as the GDPR. The definitions in the GDPR also applied to the AI Act, so, for instance, what is biometric data, but there's a whole set of new data, new concepts, for instance, the data is not only personal data. Like Shalini already said, we also have training data, validation data, testing data, input data, and we all need to be in control. We need to have mechanisms to address these things. So, the fact that the AI Act is risk-based and we now have a sort of classification of forbidden AI and high and low-risk AI already helps a bit in understanding the complexity and the possible impact on citizens. The focus of many discussions will be on classification and measures. I really applaud Clearbox AI for taking these bold steps toward working with synthetic data. I agree with everything Shalini said. Also, you can do much damage to the data when you pseudonymise or anonymise at a too early stage because the conclusions based on this poor data can be questionable, which is also a problem for research, if you do not understand what you're doing and when, and why, and so on. A lot of focus is on the data, but I think that one of the underlying problems regarding the application of AI systems is that we have two conflicting drivers: efficiency and trust. Those are things that you cannot put in algorithms so much; there’s no mathematical formula to apply. This is where the human interface is essential and human oversight and understanding the processes. We see great positive examples of this but, as earlier, also the bad examples.
What is the social impact of privacy issues in AI?
Shalini: Marlon started the interview with giving an example of the powerful social impact, what could happen to entire families, so it can be destructive both economically and health-wise, also mental health, and in terms of identity theft or reputation damage. These are things that we can see and we can probably even measure the damage, but there is also stuff that you cannot measure, like for example, manipulation, if you understand what a group of people is thinking, you can manipulate their behaviour: We know what happens with social media and the Cambridge Analytica scandal a few years ago, it was very similar. You can apply it to many different contexts. There’s also a much bigger societal impact: are we really a freedom-loving democracy as we would like to show? If we don't protect privacy, people can be manipulated and not act according to their own will.
Would you like to suggest some readings about privacy issues in AI?
Marlon: Yes, I’d suggest Hannah Arendt’s book 'The origins of totalitarianism' and some time ago I read 'Weapons of math destruction' by Cathy O'Neil, that is a very good book. Also, the Centre for Information Policy Leadership, the organization of which I am a member as well, created some time ago the so-called Accountability Wheel, so the main themes are trust and accountability. If you look up the CIPL accountability wheel, you see this framework and that's for me one of my guidelines.
Shalini: I do recognize some of the recommendations of Marlon, so I will go for a more practical implementation of what we've talked about. I think most recently I read a book, it's called 'Practical Synthetic Data Generation' and I found it very interesting. Also, it's a very practical approach about discussing all these philosophical concepts in a more applied manner. The other suggestion it's not necessarily a book but if you want to have updates especially about privacy and how professionals view privacy, I'm a part of this International Association of Privacy Professionals (IAPP). They have a lot of blogs so if you want to know about privacy and AI, there's a lot of opinion pieces from different professionals around the world about how they view it. I would recommend also checking out their blogs.
Watch the previous episodes:
Tags:
interview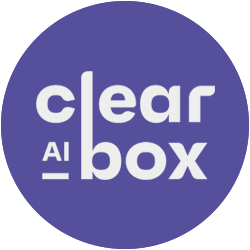