Have you ever run into Google’s ‘People also ask’ section and found the questions you were about to ask? Periodically, we are going to answer the most researched questions about AI and Machine Learning with our guests on Clearbox AI’s new interview series ‘People also Ask...and we answer’. Enjoy!
We all know that data and AI are going to rapidly transform the global economy in the next decade and beyond, but is it all about economic benefit, or can they also do good for society? In this second episode we talked about How to use data and AI for good with Shalini Kurapati, CEO at Clearbox AI, and Giovanna Jaramillo Gutierrez, Consultant Senior Epidemiologist at World Health Organization and Data Scientist at Milan and associates.
Introducing our guest
Giovanna holds a Master’s in Epidemiology and a PhD in molecular biology. She has been working as a consultant for the World Health Organization on outbreak response in the emergency program and more recently on Covid. When she’s not on a mission in field response, she also works as a data scientist in multidisciplinary teams to help companies and institutions to audit their machine learning based algorithms in high stake decisions, mostly in the health sector.
How can AI be used for good?
Shalini: AI can be used for good for a multitude of reasons, starting from what Giovanna said, in healthcare, it has immense potential in making patients’ outcomes better, in terms of predicting diseases, outbreaks and in drug discovery. So healthcare is really a fertile ground for good. In addition, we also have some lesser known aspects, like agriculture, where you can use AI to target the right amount of pesticide to the right crops; that could reduce environmental impact of chemicals, for instance. And then more and more for climate change, in terms of predicting bad weather and floods, and emergency response. The amazing part of AI is that in respect of the applications we have to think about its power, so it’s able to collect large amounts of information and give us insight so that we can make better decisions. And we can really use AI for good in pretty much every application.
Giovanna: There’s a huge opportunity here, especially in the midst of the pandemic, because we realized that there’s actually a huge amount of data being generated. So, techniques like natural language processing can be used to classify medical records for example, or we can use supervised learning to predict disease outcome based on health information datasets, and this is still a big challenge in the health sector at the moment, because of the traditional infrastructure that is a bit outdated.
What are the different types of bias in AI?
Shalini: When we talk about bias in AI, we talk about either statistical biases or racial and gender bias. Basically this comes from the fact that the datasets on which we train the AI models are not balanced enough, they are only collected from one certain part of the population. That’s also called sampling bias or representation bias. And the other aspect is historical bias, so if we train our models with older data, it means that the older societal inequalities or biases are also represented in your model. The list of AI biases can be even more than the amount of cognitive biases or biases in general, there are hundreds of them. So the main ones we deal with as of now in AI are especially the racial, inequality, gender biases, representation bias, historical bias, and sometimes even confirmation bias: that means that if the result of AI is not the same as my beliefs, I might not trust it. We could have a series of discussions on the type of biases, but these are kind of the main ones we see in AI. Therefore, these issues mostly depend on the quality of data. Like Giovanna was saying, having good quality datasets is very difficult, because the way they are collected, the way they are analysed, the way they are shared, is very different for each organisation, and you can’t really assure that everything you collect has the perfect representation, the perfect sampling etc. It’s impossible, or at least extremely complicated to get a good dataset that is balanced, that represents everybody, and it’s equitable. It’s very hard.
Giovanna: For example, a machine learning based tool is developed for a specific health problem, let’s say diagnostic, right? And, for example, the technologist will take off-the-shelf datasets that they find on Kaggle or online, and they will train the model, make a prototype and then test it, maybe deploy. But often this type of datasets don’t have metadata. What I mean by metadata is the information about those resources - where is this data coming from, what geographical region, what’s the ratio of men and women, the age, ethnicities, time of collection, because it’s very important for the disease history. For example, if it’s a laboratory sample, it’s very important to know when that sample was taken. If you use this machine learning based system in another population that was not initially reflected in the training dataset, this may lead to harmful impact in the demographic groups that, as Shalini said, are not represented in the dataset. And this may be because this dataset was taken from one country where people from that ethnicity are not represented. It’s crucial to have this discussion: data quality is the main matter and what data is used for training is also very important.
Shalini: Exactly, Giovanna. I just wanted to add another example I read recently, Even in the US the healthcare data that has been used to train AI models is collected from only three states. So it’s not representative at all of the rest of the country: even in one country they are only using it from three big states.
Giovanna: Exactly, that’s exactly what I’m referring to.
How can we integrate diversity in AI?
Giovanna: First of all, even research has shown that diverse teams of people that design these systems are really crucial, so that they can have different perspectives of the same problem definition that you want to solve. Unfortunately, even though research shows that it’s better to have diverse teams, the people that actually develop these are very narrow in terms of geography: it’s mainly in the Western world, like the US and Europe. And this means that maybe that tool will be used by people in other societies, but they are not represented, so you need to, first - in the design stage -, really have different points of view. Also, to give you a concrete example, let’s say you want to have a screening tool for breast cancer. Then it would be good to design the tool with the public health institute, the people, the patients, to have a discussion with the patients, really involve everybody across the stakeholders, because this builds trust in the tool. If you are transparent and document how it was done, what data you have used, how it was collected and so on. This is something that would be very important to have, not only one type of people that are in the decision making, but if you want this to solve the problem, you need to talk to the problem owners.
Shalini: Absolutely! Diverse teams are really an ideal solution to include diversity, but I would also like to focus on the data aspect, like you mentioned. If we want to look at a non-medical example, Wikipedia is used as a dataset for many MLOps models and also to train many types of models, and the average user of Wikipedia, the one who contributes to it, is Western, highly educated, male and white. So if you are going to train your models only on the information provided by this persona, how are you going to represent the whole world? Again, I think that diversity also comes from the importance of being aware of diversity in your datasets, like how balanced they are, and biases in your models, and also the people who are building it, I think they are all important.
Giovanna: Thank you Shalini, actually that’s really a big issue because even historically, in clinical trials, the participants were all male, adult Westerners, and there was a low awareness on the fact that we actually need more representation in clinical trials, because that data is what determines whether a treatment is effective or not, and this has led to problems. For example, when we are dealing with an AI system of a pacemaker for pregnant women but they are not included in the initial trial, this may lead to specific problems because pregnant women have more tachycardia than other patients and the device cannot interpret properly why this happens.
Shalini: Exactly! So many more examples, also in healthcare! Even the symptoms of heart attack are different for men and women, and many AI models don’t detect enough heart attack symptoms in women, there were even a few examples.
Giovanna: So this is why it’s really important to document caveats and how the models were built. It's really crucial.
What can we do to ensure good quality data?
Giovanna: So, first thank you for asking that question, because it’s a really neglected question in my view. I think most people probably don’t think it’s very sexy to talk about data quality and data collection, but I’m obsessed with data collection. I'm a field epidemiologist, that’s the core of my business and what I do. In our field, we use the expression ‘garbage in, garbage out’ to underline the importance of good quality data. This means that if your data aren’t good, the quality of your model is not going to be good. I don’t care what your performance accuracy is, I want to see what you use for training your data. I must say with the pandemic now there is more awareness in the health sector that we need good quality data in real time. We still have a big hill to go up in the sector, but we’re getting there: there’s more awareness, and I’m confident that, with the advent of the pandemic and digital health, apps and wearables are developing and they will help the growth of real time data. Specifically for developing models, there are two aspects: in the best case scenario, you can be a technologist who wants to develop machine learning based models for health and you want to work with a partner, whether it's a clinic or public health institute. They are immediately involving the core design of the data collection and you have domain experts that will tell you “yes, this dataset can be used for training: it’s valid, it’s accurate, it’s consistent, has good completeness, it has the right variables, the right timelines for data points” et cetera. What I’ve seen through my work though is more “Oh, I found this dataset online and I’m gonna use it to train my model, but I don’t know what’s the metadata, I don’t know what are the data points”. This is a very big problem because then we have to do data integration and basically a good interoperability in the health sector is not by default at all, so data integration is a big, big issue in the health sector and this is something to keep in mind. If you don’t have the right data with the right variables that make sense for the problem at hand, the problem definition and the variables you need should be very clear so that you can then evaluate the model.
Shalini: Oh yeah, I fully agree with Giovanna, data quality is really a neglected issue, also because it’s the less sexy part of doing machine learning. If you build your models and you can tweak the parameters and get better performance you get very excited, but the data quality aspect is always seen as a thankless and a manual job. You talked about metadata, Giovanna, and even adding the metadata is kind of a thankless process for some people, it’s quite manual, but it’s extremely important for the quality of the dataset. And we also know that in the whole AI cycle 80% of the time is spent on data processing and making it better, and only 20% on the models. However, if you look at the type of innovations that happen, 99% of the innovations happen on the machine learning models side, and very little on the data quality side. Another aspect I would also like to mention in addition to data quality: there are a lot of concerns regarding privacy, for instance. So when you collect the data, if I have to build a machine learning model, how can we use this in a way that protects the rights of the individuals as well, so when you are building AI models it’s also very hard to assure them that the risk of re-identification is low. Actually right now, even at Clearbox AI, we are thinking more on a data-centric perspective, and for instance we are now using techniques like synthetic data to start building models where we can not only assess the data more efficiently, but also augment it and try to see if there are imbalances. Can we have synthetic data that can be more representative than the original one? Can we reduce biases in synthetic data? So right now we are also having use cases for privacy preservation but it’s only one of the ways to block the issues we see nowadays.
If you want to know more about how Explainable AI helps the use of data and AI for good, check out our previous episode here!
Tags:
interview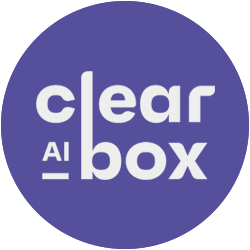