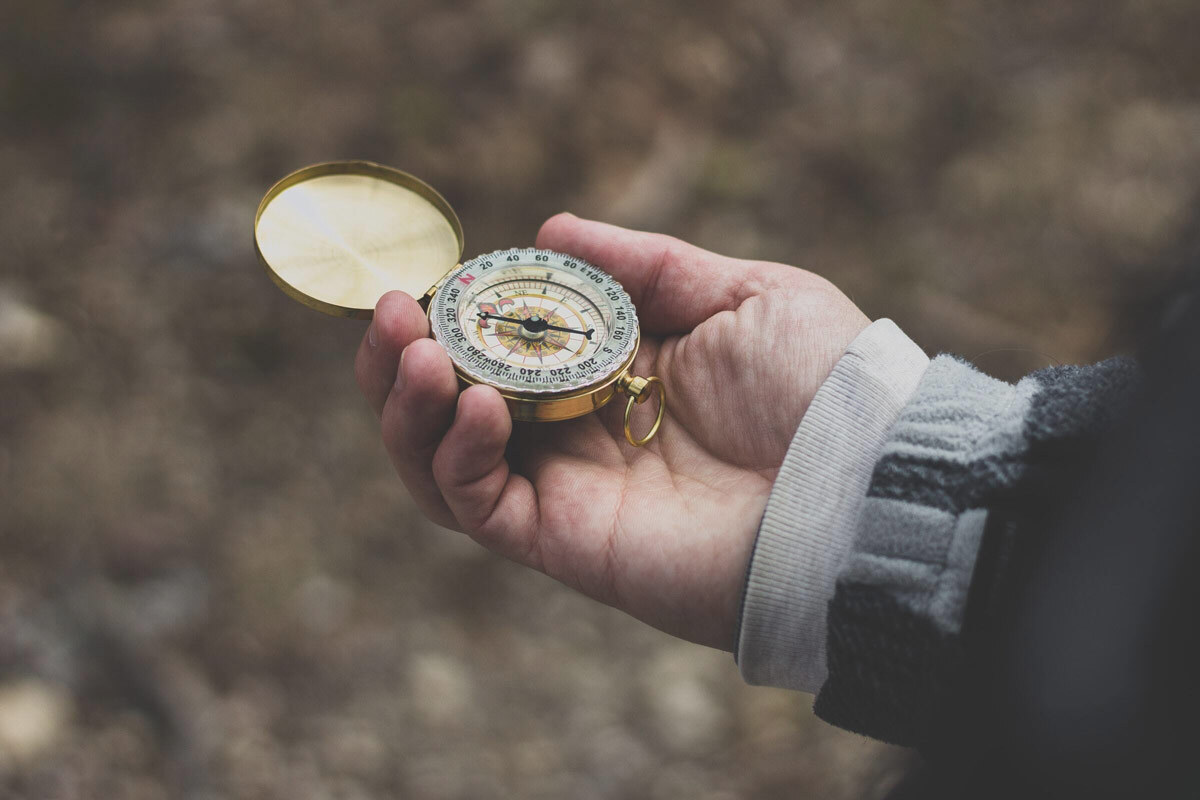
On 3 and 4 Dec, 2020, I had the wonderful opportunity to participate in the Openness and Commercialisation conference (#OSBiz2020) co-organised by CESAER and various leading universities of technologies across Europe and the UK. The conference dealt with complex and often understated aspects of balancing the openness of innovation while sustaining commercial aspirations for the same.
I was also invited as a speaker to take part in a panel that highlights how companies view these aspects. Together with colleagues from AstraZeneca, Siemens and AST we had fruitful discussion on how to achieve the balance between openness and commercialisation. The overall panel discussion has been comprehensively reported by Mattias Björnmalm and Yvonne Kinnard, in this Cesaer article. Some parts of this current post have been adapted from their notes. Also, Connie Claire from TU Delft neatly illustrated the overall conference highlights in this article.
In the panel discussion with industry colleagues, I represented the perspective of an up and coming AI technology startup with a niche product, our AI model assessment tool. It is a value added product that plug-into for MLops pipelines. It helps companies to assess, monitor and improve their AI models to pave the way for trustworthy AI adoption at scale.
I couldn’t have asked for a more relevant panel to be part of! Here are some of the highlights of my contribution from the perspective of Clearbox AI.
AI touches upon all facets of today’s innovation landscape. Its application in business is relatively new. There is a strong culture of industry-academia collaboration in this field due to the need for sustained R&D efforts to hone and apply it in the most effective manner possible. The lines between research labs and business units practicing AI are really blurry. Companies, including startups like Clearbox AI, that develop AI products are expected to demonstrate their technical excellence and publish papers, often with academic partners. The immense excitement and buzz around AI innovation is justifiable as it is a powerful tool that can (positively) impact the way we work and live.
Notwithstanding the excitement, there is always a palpable level of apprehension associated with AI. Most powerful AI models are black boxes: as long as you give them tons of data, they can be great at predictions, decision making or giving recommendations. We cannot blindly trust these models at face value and we don’t have straightforward methods to understand, assess and control these models. There are a number of ethics, fairness and regulatory issues that come along with this, but the core problem is trust. While most companies want to innovate with AI, a fraction of them are able to do so within their business operations. Arguably, AI’s blackbox problem is the main cause for the said trust issues in AI. Another reason for the slow AI adoption can be attributed to low reproducibility/replication of AI research studies/papers. i.e. practitioners and researchers often have difficulties in reproducing the findings of other researchers. This further lowers trust in AI. AI can benefit a lot from openness, but we need to define what openness is. For example in the same conference Prof. Alan Hansbury talked about openness with closed data. AI often falls in that domain with high volume, dynamic and personal data. So we need to figure out ways to increase trust and reproducibility, so that companies can actually put AI models into production in a trustworthy and responsible way.
Within the definition of openness for AI we need to consider the robustness, validation and reproducibility aspects. One example that we discussed with Sophie of AstraZeneca, was about AI models for diagnostics which worked beautifully in one hospital but failed completely when deployed in another. A likely reason is minor differences in the equipment and settings used, which is hard to predict beforehand, but of course crucial for real-life usage.
When it comes to real-world applications of AI, the concept of openness can be complicated. Raw data is often very dirty and processing, curating and maintaining data sets is therefore very costly. Open source tools need dedicated community support and following. They may not always work, sometimes can create a lot of headaches for developers to build and sustain their workflow using these tools. Bottomline: Openness, however you want to define it, cannot always be free.
We need to approach openness in terms of trust, reproducibility and interoperability. That is when we have the most productive discussions on openness while implementing new technologies like Artificial Intelligence.
Tags:
blogpost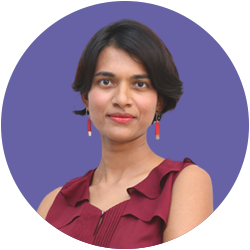